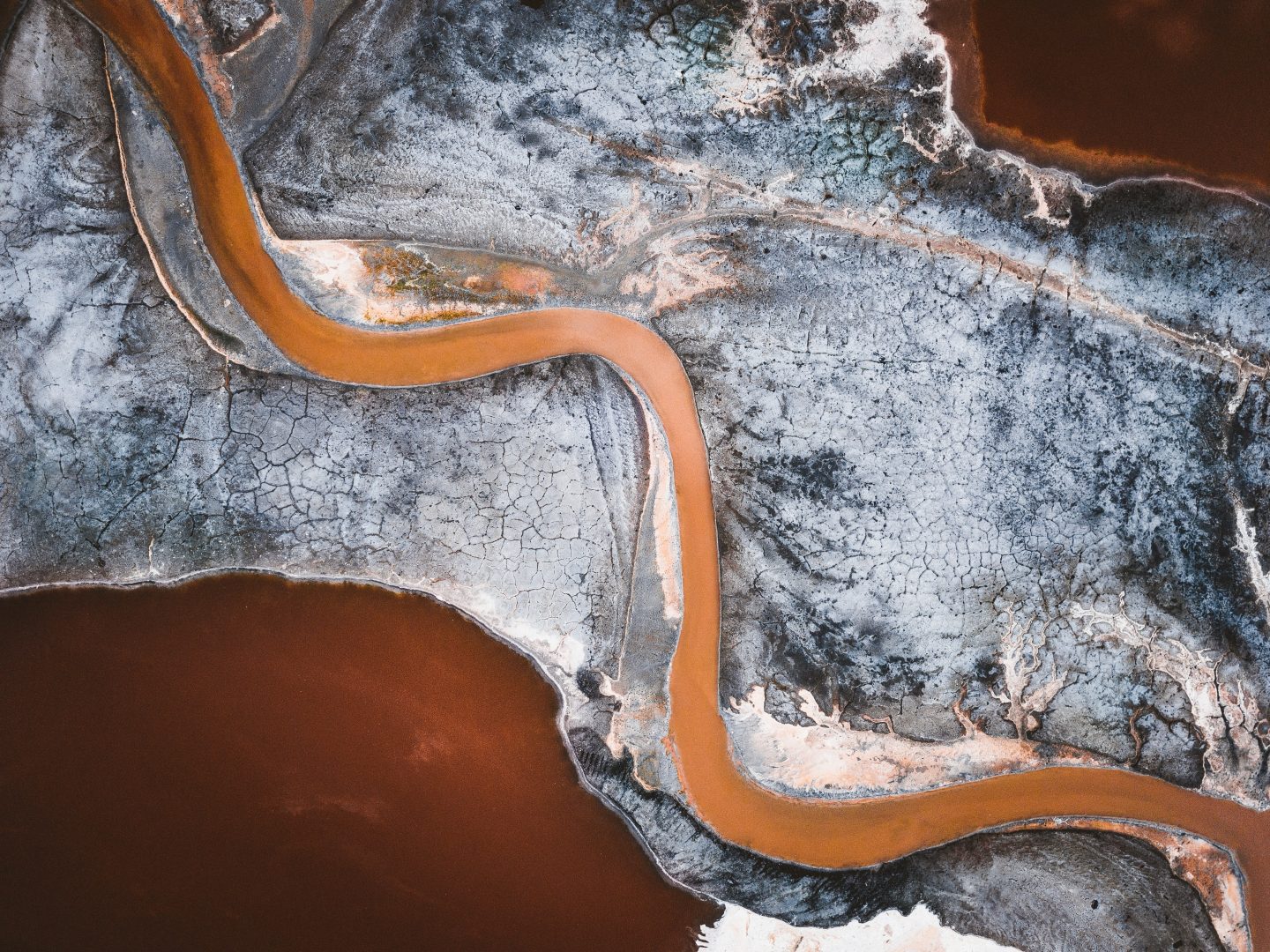
Controlling Contamination Bias in Child Maltreatment Research
Controlling Contamination Bias in Child Maltreatment Research
Contamination occurs in many different experimental designs outside the field of child maltreatment. In this project, contamination refers to the presence of child maltreatment in already established, non-child maltreatment comparison conditions. Research has shown that failure to detect and control contamination biases effect size estimates for child maltreatment outcomes and leads to variation in the significance and magnitude of those estimates within and across studies, increasing the chances of discovery and replication failures. The Detecting and Controlling Contamination Bias
Project (Shenk, PI; Shores, Ram, & Fisher Co-I’s) is supported by awards from the National Institutes of Health (R03HD104739) and the National Science Foundation (BCS-2041333) examining contamination in prospective cohort studies of child maltreatment. The current project is using existing data from two large, multi-wave, prospective cohort studies of confirmed child maltreatment, the Longitudinal Studies of Child Abuse and Neglect (LONGSCAN; N=1354) and the National Survey of Child and Adolescent Well-being-II (NSCAW-II; N=5872), to accomplish two specific aims: 1) estimate the prevalence of contamination, defined as any self- or caregiver-reported instance of child maltreatment by members of the established comparison condition, and 2) test different statistical approaches for reducing bias in risk estimates for child behavior problems attributable to contamination. Finally, this project will conduct extensive data simulations based on these results to extend inferences across different research conditions, including variations in sample size, contamination prevalence, statistical power, and effect size magnitude. The end product of this project will be to disseminate to the larger scientific field the optimal methods for detecting and controlling contamination bias across a range of research conditions in order to minimize variation in the significance and magnitude of effect size estimates reported across prospective studies.
T32 Fellows will have the opportunity to generate, execute, and report results from statistical models aiming to establish the optimal detection and control of contamination. Statistical models where Fellows can receive training include, multi-level modeling, propensity score matching, and synthetic controls.